Identify threats to data integrity
For data to retain its value and health, it must be complete, correct, relevant, and up-to-date. There are various factors that exist within your organization that compromise the integrity of your data, including outdated software, negligent employees, manual processes, and inconsistent practices.
Negligent staff requires coaching, but careless human error is bound to happen again without defined processes or quality control measures in place. Manual processes are also drivers for human error because manual data entry is tedious – increasing the risk of careless mistakes. Having non-standard data requirements also leads to inconsistencies, bottlenecks, and automation disruption.
Map out all data processes
From collecting data to maintaining databases, defining and documenting process maps for all data-related activities helps organizations gain better control of their data. The documentation gives employees something to refer to when there are questions about gathering or maintaining databases. Having data-related processes outlined and documented also improves training retention.
Beyond regular cleaning of duplicate and incomplete data, organizations should ensure they’re not holding onto outdated data. Your company stakeholders’ data will change; customers, clients, investors, employees, suppliers, and vendors may move addresses, change positions and titles, or leave altogether. Therefore, not anticipating these changes may lead to a database riddled with unusable addresses and irrelevant contacts. Therefore, a process for preventing outdated data should include a defined and regular schedule.
Standardize contact data
One of the main culprits of data inconsistencies is the lack of data standardization. Employees come from different educational and professional backgrounds. Each person may have their own way of inputting names, addresses, etc., for example, using “st.” vs. spelling out “street” or spelling out dates vs. its numerical form. The differences may seem trivial but can be enough to slow down systems and people, affecting how teams perform searches and extract data. Without a defined standard, collected data can harm processes, personalization, the validity of analytics, and automation.
Implement quality control measures
Maintain data integrity by having a validation process in place, cleaning data regularly, and backing up data. To ensure the quality of the data, databases should be cleansed often by removing duplicate data and validating the accuracy of the data. Leverage integration tools that allow you to detect and remove errors and inconsistencies automatically. The goal should be to reduce manual inspection processes that may only increase the risk of error.
To learn how we can help you and your business, complete the contact form.
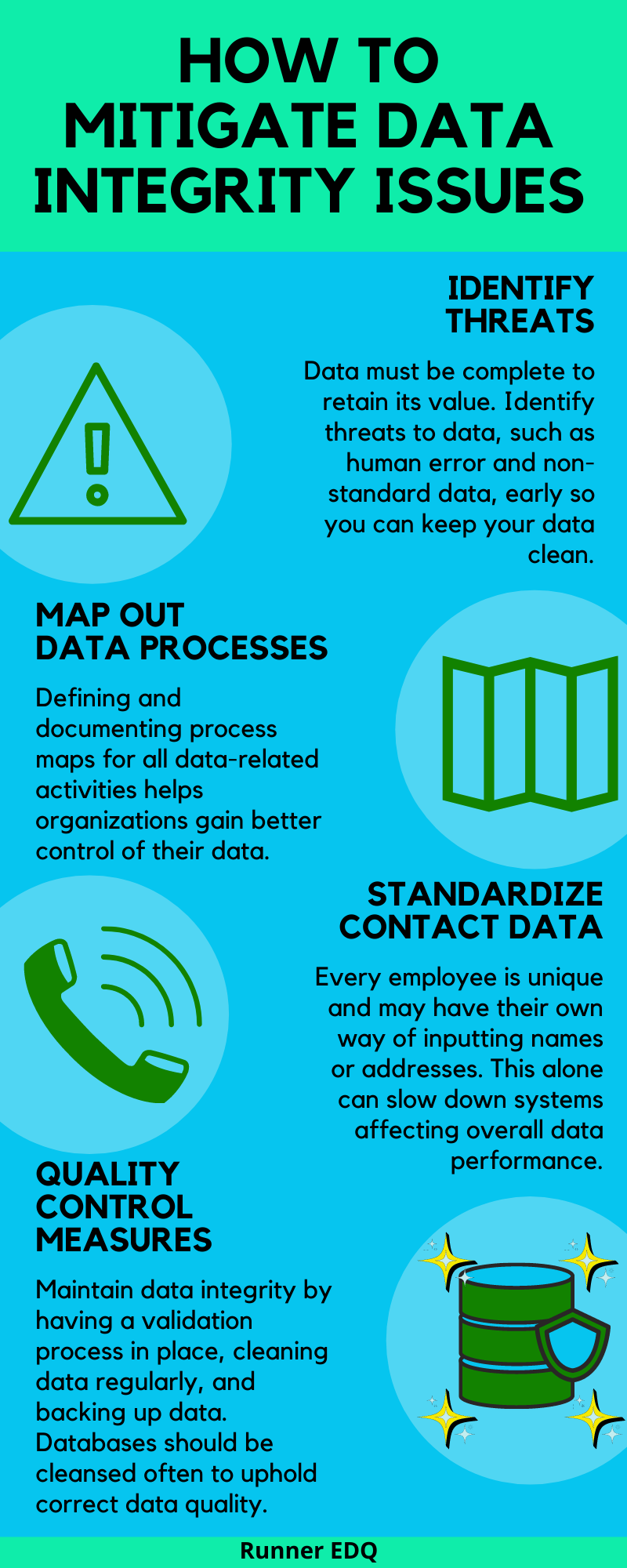